Date d'évènement : 29/04/2025
Antoine Benady - Laboratoire de Mécanique Paris-Saclay
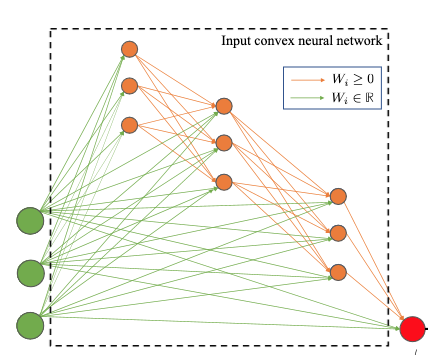
Unsupervised strategies for the learning of constitutive model with neural networks
The use of neural networks (NNs) to learn mechanical constitutive models is a very active area of research. Representing constitutive models in a thermodynamically consistent manner with NNs has become a well-established approach. These networks can be trained using measurable data within unsupervised frameworks, such as NN-EUCLID (see [1] for the original article and [2] for experimental validation) or NN-mCRE [3].
The aim of this talk is to present recent advances in unsupervised learning applied to the modeling of mechanical constitutive laws. In particular, the two previously mentioned unsupervised approaches will be compared with respect to criteria such as computational efficiency, noise robustness, type of data required, and sensitivity to initialization.
References
[1] P. Thakolkaran, A. Joshi, Y. Zheng, M. Flaschel, L. De Lorenzis, S. Kumar. NN-EUCLID: Deep-learning hyperelasticity without stress data. Journal of the Mechanics and Physics of Solids (2022).
[2] C. Jailin, A. Benady, R. Legroux, E. Baranger. Experimental Learning of a Hyperelastic Behavior with a Physics-Augmented Neural Network. Experimental Mechanics (2024)
[3] A. Benady, E. Baranger, L. Chamoin. NN-mCRE: A modified constitutive relation error framework for unsupervised learning of nonlinear state laws with physics-augmented neural networks. Int J Numer. Methods Eng. (2024).
![]() |
‼️ Le séminaire peut être suivi en ligne suivant le lien : https://univ-amu-fr.zoom.us/j/95940389321?pwd=jaGSbcDLo2abMGXhQbA2cAW2GRzCeU.1 |
Le mardi 29 avril 2025 à 11h00 / Amphithéâtre François Canac, LMA
Voir en ligne : plus d’informations concernant l’orateur